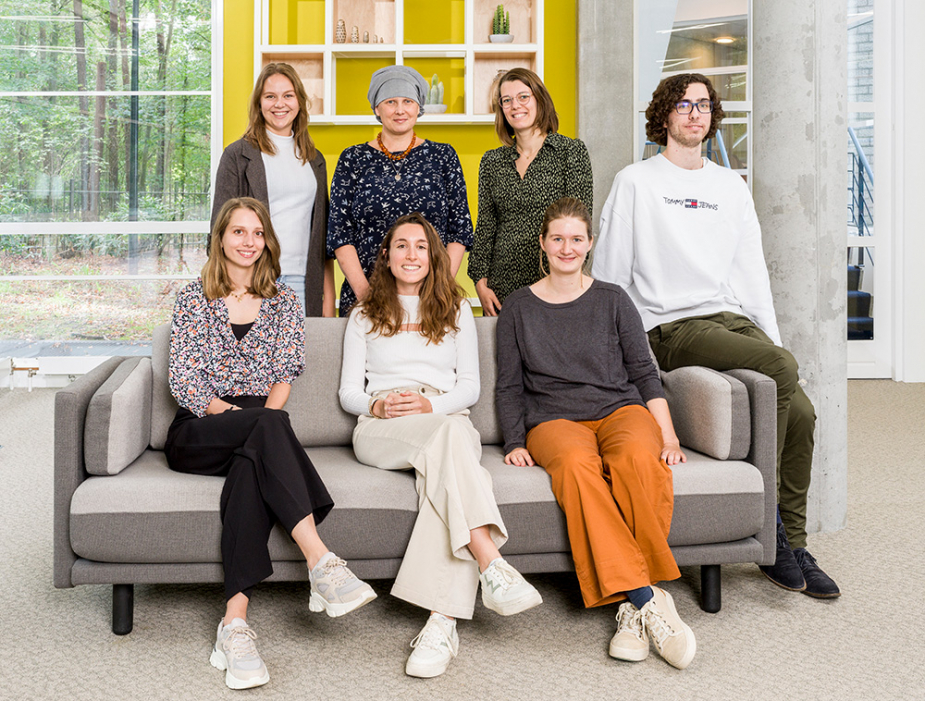
Group leader
Post doc
PhD students
MSc students
Pedro Alonso Gonzalez
Julia Niehaus
Research assistant
Student assistant
Rafael Zampakas
Alumni
Chin Yang Shapland
Marjolein van Donkelaar
Mariska Barendse
Laurence Howe (co-supervision with University of Bristol, Professor George Davey Smith and Dr Sarah Lewis)
Janne Vermeulen
Jeffrey van der Ven
Mitchell Olislagers
Celeste Figaroa
Tanguy Rubat du Mérac
Simone van den Bedem
Fenja Schlag
Anh Nguyen
Paola Moreno Ancalmo
Displaying 261 - 280 of 15536
-
Woensdregt, M., Blokpoel, M., Van Rooij, I., & Martin, A. E. (2024). Challenges for a computational explanation of flexible linguistic inference. Poster presented at the 46th Annual Meeting of the Cognitive Science Society (CogSci 2024), Rotterdam, The Netherlands.
-
Liesenfeld, A., & Dingemanse, M. (2024). Interactive probes: Towards action-level evaluation for dialogue systems. Discourse & Communication, 18(6), 954-964. doi:10.1177/17504813241267071.
Abstract
Measures of ‘humanness’, ‘coherence’ or ‘fluency’ are the mainstay of dialogue system evaluation, but they don’t target system capabilities and rarely offer actionable feedback. Reviewing recent work in this domain, we identify an opportunity for evaluation at the level of action sequences, rather than the more commonly targeted levels of whole conversations or single responses. We introduce interactive probes, an evaluation framework inspired by empirical work on social interaction that can help to systematically probe the capabilities of dialogue systems. We sketch some first probes in the domains of tellings and repair, two sequence types ubiquitous in human interaction and challenging for dialogue systems. We argue interactive probing can offer the requisite flexibility to keep up with developments in interactive language technologies and do justice to the open-endedness of action formation and ascription in interaction. -
Bauer, B. L. M. (2024). Counting systems: Decimalization and linguistic complexity. Talk presented at the 21st International Congress of Linguists (ICL). Poznań, Poland. 2024-09-08 - 2024-09-14.
-
Basile, G. A., Nozais, V., Quartarone, A., Giustiniani, A., Ielo, A., Cerasa, A., Milardi, D., Abdallah, M., Thiebaut de Schotten, M., Forkel, S. J., & Cacciola, A. (2024). Functional anatomy and topographical organization of the frontotemporal arcuate fasciculus. Communications Biology, 7: 1655. doi:10.1038/s42003-024-07274-3.
Abstract
Traditionally, the frontotemporal arcuate fasciculus (AF) is viewed as a single entity in anatomo-clinical models. However, it is unclear if distinct cortical origin and termination patterns within this bundle correspond to specific language functions. We use track-weighted dynamic functional connectivity, a hybrid imaging technique, to study the AF structure and function in two distinct datasets of healthy subjects. Here we show that the AF can be subdivided based on dynamic changes in functional connectivity at the streamline endpoints. An unsupervised parcellation algorithm reveals spatially segregated subunits, which are then functionally quantified through meta-analysis. This approach identifies three distinct clusters within the AF - ventral, middle, and dorsal frontotemporal AF - each linked to different frontal and temporal termination regions and likely involved in various language production and comprehension aspects. Our findings may have relevant implications for the understanding of the functional anatomy of the AF as well as its contribution to linguistic and non-linguistic functions.Additional information
supplementary information -
Koning, M. E. E., Wyman, N. K., Menks, W. M., Ekerdt, C., Fernández, G., Kidd, E., Lemhöfer, K., McQueen, J. M., & Janzen, G. (2024). The relationship between brain structure and function during novel grammar learning across development. Cerebral Cortex, 34(12): bhae488. doi:10.1093/cercor/bhae488.
Abstract
In this study, we explored the relationship between developmental differences in gray matter structure and grammar learning ability in 159 Dutch-speaking individuals (8 to 25 yr). The data were collected as part of a recent large-scale functional MRI study (Menks WM, Ekerdt C, Lemhöfer K, Kidd E, Fernández G, McQueen JM, Janzen G. Developmental changes in brain activation during novel grammar learning in 8–25-year-olds. Dev Cogn Neurosci. 2024;66:101347. https://doi.org/10.1016/j.dcn.2024.101347) in which participants implicitly learned Icelandic morphosyntactic rules and performed a grammaticality judgment task in the scanner. Behaviorally, Menks et al. (2024) showed that grammaticality judgment task performance increased steadily from 8 to 15.4 yr, after which age had no further effect. We show in the current study that this age-related grammaticality judgment task performance was negatively related to cortical gray matter volume and cortical thickness in many clusters throughout the brain. Hippocampal volume was positively related to age-related grammaticality judgment task performance and L2 (English) vocabulary knowledge. Furthermore, we found that grammaticality judgment task performance, L2 grammar proficiency, and L2 vocabulary knowledge were positively related to gray matter maturation within parietal regions, overlapping with the functional MRI clusters that were reported previously in Menks et al. (2024) and which showed increased brain activation in relation to grammar learning. We propose that this overlap in functional and structural results indicates that brain maturation in parietal regions plays an important role in second language learning.Additional information
supplements -
Dalla Bella, S., Janaqi, S., Benoit, C.-E., Farrugia, N., Bégel, V., Verga, L., Harding, E. E., & Kotz, S. A. (2024). Unravelling individual rhythmic abilities using machine learning. Scientific Reports, 14(1): 1135. doi:10.1038/s41598-024-51257-7.
Abstract
Humans can easily extract the rhythm of a complex sound, like music, and move to its regular beat, like in dance. These abilities are modulated by musical training and vary significantly in untrained individuals. The causes of this variability are multidimensional and typically hard to grasp in single tasks. To date we lack a comprehensive model capturing the rhythmic fingerprints of both musicians and non-musicians. Here we harnessed machine learning to extract a parsimonious model of rhythmic abilities, based on behavioral testing (with perceptual and motor tasks) of individuals with and without formal musical training (n = 79). We demonstrate that variability in rhythmic abilities and their link with formal and informal music experience can be successfully captured by profiles including a minimal set of behavioral measures. These findings highlight that machine learning techniques can be employed successfully to distill profiles of rhythmic abilities, and ultimately shed light on individual variability and its relationship with both formal musical training and informal musical experiences.Additional information
supplementary materials -
Koutamanis, E., Kootstra, G. J., Dijkstra, T., & Unsworth, S. (2024). Cognate facilitation in single- and dual-language contexts in bilingual children’s word processing. Linguistic Approaches to Bilingualism, 14(4), 577-608. doi:10.1075/lab.23009.kou.
Abstract
We examined the extent to which cognate facilitation effects occurred in simultaneous bilingual children’s production and comprehension and how these were modulated by language dominance and language context. Bilingual Dutch-German children, ranging from Dutch-dominant to German-dominant, performed picture naming and auditory lexical decision tasks in single-language and dual-language contexts. Language context was manipulated with respect to the language of communication (with the experimenter and in instructional videos) and by means of proficiency tasks. Cognate facilitation effects emerged in both production and comprehension and interacted with both dominance and context. In a single-language context, stronger cognate facilitation effects were found for picture naming in children’s less dominant language, in line with previous studies on individual differences in lexical activation. In the dual-language context, this pattern was reversed, suggesting inhibition of the dominant language at the decision level. Similar effects were observed in lexical decision. These findings provide evidence for an integrated bilingual lexicon in simultaneous bilingual children and shed more light on the complex interplay between lexicon-internal and lexicon-external factors modulating the extent of lexical cross-linguistic influence more generally. -
Eising, E. (2024). Monogenic contributions to speech delay. Talk presented at the CRE Symposium ‘What’s new since FOXP2: new developments in speech and language neurobiology’. London. 2024-06-07.
-
Schulz, F. M., Corps, R. E., & Meyer, A. S. (2024). Who is a fluent speaker? Working memory might tell us!. Poster presented at the IMPRS Conference 2024, Nijmegen, the Netherlands.
-
Yang, J. (2024). Rethinking tokenization: Crafting better tokenizers for large language models. International Journal of Chinese Linguistics, 11(1), 94-109. doi:10.1075/ijchl.00023.yan.
Abstract
Tokenization significantly influences language models (LMs)’ performance. This paper traces the evolution of tokenizers from word-level to subword-level, analyzing how they balance tokens and types to enhance model adaptability while controlling complexity. Despite subword tokenizers like Byte Pair Encoding (BPE) overcoming many word tokenizer limitations, they encounter difficulties in handling non-Latin languages and depend heavily on extensive training data and computational resources to grasp the nuances of multiword expressions (MWEs). This article argues that tokenizers, more than mere technical tools, should drawing inspiration from the cognitive science about human language processing. This study then introduces the “Principle of Least Effort” from cognitive science, that humans naturally seek to reduce cognitive effort, and discusses the benefits of this principle for tokenizer development. Based on this principle, the paper proposes that the Less-is-Better (LiB) model could be a new approach for LLM tokenizer. The LiB model can autonomously learn an integrated vocabulary consisting of subwords, words, and MWEs, which effectively reduces both the numbers of tokens and types. Comparative evaluations show that the LiB tokenizer outperforms existing word and BPE tokenizers, presenting an innovative method for tokenizer development, and hinting at the possibility of future cognitive science-based tokenizers being more efficient. -
Wong, M. M. K., Sha, Z., Luetje, L., Kong, X., Velthuijs, N., Van Heukelum, S., Schijven, D., Mhlanga, M., Van de Berg, W., Jonkman, L., Fisher, S. E., & Francks, C. (2024). The neocortical infrastructure for language involves region-specific patterns of laminar gene expression. Poster presented at the Highlights in the Language Sciences Conference 2024, Nijmegen, The Netherlands.
-
Huettig, F., & Christiansen, M. H. (2024). Can large language models counter the recent decline in literacy levels? An important role for cognitive science. Cognitive Science, 48(8): e13487. doi:10.1111/cogs.13487.
Abstract
Literacy is in decline in many parts of the world, accompanied by drops in associated cognitive skills (including IQ) and an increasing susceptibility to fake news. It is possible that the recent explosive growth and widespread deployment of Large Language Models (LLMs) might exacerbate this trend, but there is also a chance that LLMs can help turn things around. We argue that cognitive science is ideally suited to help steer future literacy development in the right direction by challenging and informing current educational practices and policy. Cognitive scientists have the right interdisciplinary skills to study, analyze, evaluate, and change LLMs to facilitate their critical use, to encourage turn-taking that promotes rather than hinders literacy, to support literacy acquisition in diverse and equitable ways, and to scaffold potential future changes in what it means to be literate. We urge cognitive scientists to take up this mantle—the future impact of LLMs on human literacy skills is too important to be left to the large, predominately US-based tech companies. -
Slaats, S. (2024). On the interplay between lexical probability and syntactic structure in language comprehension. PhD Thesis, Radboud University Nijmegen, Nijmegen.
Additional information
full text via Radboud Repository -
Dang, A., Raviv, L., & Galke, L. (2024). Morphology matters: Probing the cross-linguistic morphological generalization abilities of large language models through a Wug Test. In CMCL 2024 - 13th Edition of the Workshop on Cognitive Modeling and Computational Linguistics, Proceedings of the Workshop (pp. 177-188). Kerrville, TX, USA: Association for Computational Linguistics (ACL).
-
Moser, C., Tarakçı, B., Ünal, E., & Grigoroglou, M. (2024). Multimodal Description of Instrument Events in Turkish and English. In L. K. Samuelson, S. L. Frank, A. Mackey, & E. Hazeltine (
Eds. ), Proceedings of the 46th Annual Meeting of the Cognitive Science Society (CogSci 2024) (pp. 341-348).Abstract
Daily experiences are conceptualized as events involving multiple participants and their relations (i.e., thematic roles). When describing events, speakers often do not include all event participants involved. Here, we explore how underlying conceptual requirements and language-specific encoding options influence the content of event descriptions in speech and gesture in two typologically different languages (English,
Turkish). Focusing on conceptually peripheral instruments whose status is highly debated, we manipulated the conceptual status of event participants by including events that ‘require’ or ‘allow’ otherwise syntactically optional instruments. Results showed that the require-allow distinction did not manifest uniformly in Turkish and English in speech, gesture, or when both modalities were considered. However, mention of highly optional event participants (e.g., allowed instruments) was
affected by language-specific syntactic encoding options. We conclude that, under more naturalistic elicitation conditions, planning descriptions of instrument events is more heavily affected by language-specific encoding than conceptual prominence of the roles.Additional information
https://escholarship.org/uc/item/31h4s3qp -
Verhoef, E., De Hoyos, L., Schlag, F., Van der Ven, J., Olislagers, M., Dale, P., Kidd, E., Fisher, S. E., & St Pourcain, B. (2024). Developing language in a developing body: Genetic associations of infant motor and personal-social skills with emerging language abilities. PsyArXiv Preprints. doi:10.31234/osf.io/6mtd8.
Abstract
Mastering developmental milestones such as infant motor and personal-social skills (including social routines and pretend-play) can initiate a cascade of developmental changes that may affect language learning. Specifically, motor development may represent an important, but little understood “gateway” enabling children to interact with their environment. Here, we investigate how infant motor and personal-social abilities link to infant and toddler language performance, using a genetic perspective. For this, we studied measures of motor and personal-social skills (6 and 15 months) as predictors of language development, captured by ten language phenotypes (15-38 months) in genotyped children from the Avon Longitudinal Study of Parents and Children (N≤7,017). Language measures were combined into language factor scores (LFS) using structural equation modelling. Developmental genomic and non-genomic (residual) relationships across phenotypes were modelled with a Cholesky decomposition using genetic-relationship-matrix structural equation modelling (GRM-SEM). The ten early language measures were captured by three interrelated language factors (F-15M, F-24M, F-38M, 51.3% explained variance), each corresponding to a different age window. Across infant predictors and derived language factor scores, common genetic variation accounted for a modest proportion of the phenotypic variance (known as heritability, h2: gross-motor-abilities-6M-h2=0.18(SE=0.06), personal-social-skills-15M-h2=0.18(SE=0.06), LFS-15M-h2=0.12(SE=0.05), LFS-24M-h2=0.21(SE=0.06), LFS-38M-h2=0.17(SE=0.05)). Fitting a Cholesky GRM-SEM across predictors and LFS showed that infant gross motor abilities shared genetic influences with personal-social skills (factor loading λ; personal-social-skills-15M-λ=0.22(SE=0.09)), but were unrelated to language performance (P≥0.05). In contrast, genetic influences underlying personal-social skills, independent of gross motor skills, were related to all three LFS (LFS-15M-λ=0.26(SE=0.09), LFS-24M-λ=0.28(SE=0.10), LFS-38M-λ=0.30(SE=0.10)). GRM-SEM analyses studying individual language outcomes provided consistent results, both for genomic and non-genomic structures. Thus, aetiological processes linking motor to personal-social skills differ from those linking personal-social to language abilities, consistent with a developmental cascade where motor control enables children to engage in novel social interactions, but children’s social learning abilities foster language development.Additional information
supplementary materials -
Holler, J. (2024). Producing and comprehending multimodal utterances in face-to-face conversation [keynote]. Talk presented at the 2nd International Multimodal Communication Symposium (MMSYM 2024). Frankfurt, Germany. 2024-09-25 - 2024-09-27.
-
Arana, S., Pesnot Lerousseau, J., & Hagoort, P. (2024). Deep learning models to study sentence comprehension in the human brain. Language, Cognition and Neuroscience, 39(8), 972-990. doi:10.1080/23273798.2023.2198245.
Abstract
Recent artificial neural networks that process natural language achieve unprecedented performance in tasks requiring sentence-level understanding. As such, they could be interesting models of the integration of linguistic information in the human brain. We review works that compare these artificial language models with human brain activity and we assess the extent to which this approach has improved our understanding of the neural processes involved in natural language comprehension. Two main results emerge. First, the neural representation of word meaning aligns with the context-dependent, dense word vectors used by the artificial neural networks. Second, the processing hierarchy that emerges within artificial neural networks broadly matches the brain, but is surprisingly inconsistent across studies. We discuss current challenges in establishing artificial neural networks as process models of natural language comprehension. We suggest exploiting the highly structured representational geometry of artificial neural networks when mapping representations to brain data.Additional information
link to preprint -
Bianco, R., Zuk, N. J., Bigand, F., Quarta, E., Grasso, S., Arnese, F., Ravignani, A., Battaglia-Mayer, A., & Novembre, G. (2024). Neural encoding of musical expectations in a non-human primate. Current Biology, 34(2), 444-450. doi:10.1016/j.cub.2023.12.019.
Abstract
The appreciation of music is a universal trait of humankind.1,2,3 Evidence supporting this notion includes the ubiquity of music across cultures4,5,6,7 and the natural predisposition toward music that humans display early in development.8,9,10 Are we musical animals because of species-specific predispositions? This question cannot be answered by relying on cross-cultural or developmental studies alone, as these cannot rule out enculturation.11 Instead, it calls for cross-species experiments testing whether homologous neural mechanisms underlying music perception are present in non-human primates. We present music to two rhesus monkeys, reared without musical exposure, while recording electroencephalography (EEG) and pupillometry. Monkeys exhibit higher engagement and neural encoding of expectations based on the previously seeded musical context when passively listening to real music as opposed to shuffled controls. We then compare human and monkey neural responses to the same stimuli and find a species-dependent contribution of two fundamental musical features—pitch and timing12—in generating expectations: while timing- and pitch-based expectations13 are similarly weighted in humans, monkeys rely on timing rather than pitch. Together, these results shed light on the phylogeny of music perception. They highlight monkeys’ capacity for processing temporal structures beyond plain acoustic processing, and they identify a species-dependent contribution of time- and pitch-related features to the neural encoding of musical expectations. -
Dikshit, A. P., Das, D., Samal, R. R., Parashar, K., Mishra, C., & Parashar, S. (2024). Optimization of (Ba1-xCax)(Ti0.9Sn0.1)O3 ceramics in X-band using Machine Learning. Journal of Alloys and Compounds, 982: 173797. doi:10.1016/j.jallcom.2024.173797.
Abstract
Developing efficient electromagnetic interference shielding materials has become significantly important in present times. This paper reports a series of (Ba1-xCax)(Ti0.9Sn0.1)O3 (BCTS) ((x =0, 0.01, 0.05, & 0.1)ceramics synthesized by conventional method which were studied for electromagnetic interference shielding (EMI) applications in X-band (8-12.4 GHz). EMI shielding properties and all S parameters (S11 & S12) of BCTS ceramic pellets were measured in the frequency range (8-12.4 GHz) using a Vector Network Analyser (VNA). The BCTS ceramic pellets for x = 0.05 showed maximum total effective shielding of 46 dB indicating good shielding behaviour for high-frequency applications. However, the development of lead-free ceramics with different concentrations usually requires iterative experiments resulting in, longer development cycles and higher costs. To address this, we used a machine learning (ML) strategy to predict the EMI shielding for different concentrations and experimentally verify the concentration predicted to give the best EMI shielding. The ML model predicted BCTS ceramics with concentration (x = 0.06, 0.07, 0.08, and 0.09) to have higher shielding values. On experimental verification, a shielding value of 58 dB was obtained for x = 0.08, which was significantly higher than what was obtained experimentally before applying the ML approach. Our results show the potential of using ML in accelerating the process of optimal material development, reducing the need for repeated experimental measures significantly.
Share this page